Unlocking your data capability
Tim Cobbett
Tim Cobbett has worked in registry and planning roles across six HEIs, along with a 3-year spell working as a UUK Policy Adviser. His consultancy work has covered student systems; administrative processes; data management; admissions; metrics and reporting; student dispute management; and academic governance.
If you put “data” into Google you get 8.97 billion results. For comparison, “Covid” gets 6.55 billion, “Trump” gets 1.78 billion, “Brexit” gets 182 million. There are many reasons why data has become big, not least of which is the development of technologies and protocols to capture data easily, store vast amounts of it cheaply, andretrieve it quickly. In addition it is now much easier to store, combine and analyse multiple datasets, leading to the potential for new correlations and insights. At the same time, the uses to which data can be put have multiplied and become more powerful: in addition to business intelligence and conventional statistical analysis we now have data analytics, clustering, machine learning algorithms, and artificial intelligence.
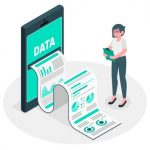
It is not surprising that higher education providers often aspire to make better use of their data. After all, most HEPS are sitting on a lot of it, including detailed personal data, attendance and other time-space data, achievement data, relatively granular financial data, etc. And some of the benefits of using this data are easy to perceive, like a more efficient funnel for student recruitment, more effective strategies for student learning at both individual and collective level, robust bottom-up activity costings.
However, I think there are a few problems to overcome. As an HE consultant I keep coming across universities and colleges that are still struggling to do the ‘traditional’ stuff with their data. The data for simple reports is difficult to extract, and the reports are seen as unreliable and challengeable. The department(s) responsible for the HESA return(s) engage in the same painful struggle every year. There are difficulties in reconciling statutory and internal reporting. I therefore have some scepticism about the ability of these institutions to move to the world of integrated datasets and sophisticated data analysis. (Although my viewpoint is inevitably skewed by the fact that I don’t normally get asked as a consultant to advise on things that are already working well!).
There are a number of underlying issues that can cause these problems. Long-standing core systems (particularly student systems) may have evolved idiosyncrasies and only be understood by a very small number of people. This is often compounded by the existence of multiple local datasets, leading to a situation where there is no single source of truth. Another common problem is differing definitions of key data items, and a lack of cross-institutional knowledge of how, why and when data is collected, derived and used.
Fixing these data basics is essential, not just for the traditional reports but in order to provide a foundation for the exciting new stuff. Indeed, it’s even more essential when you start using multi-datasets, analytics, machine learning algorithms and the like, because the outputs of these methods are notoriously opaque. It’s relatively easy to identify issues with numbers, percentages or different types of average; it’s much harder to identify underlying errors in, say, a forecast based on multiple linear regression, particularly when it comes in the form of a fancy graphic.
So what practical steps might a university take towards better use of data? I’d like to suggest three areas to focus, under the broad heading of robust data governance. This includes:
1. Data definition
Putting in place high level definitions (e.g. what is an “international student”), detailed data dictionaries for core datasets, data lineage (where data items come from, how they are used, how they relate to other data items including those in different datasets). It is also important to define ownership of datasets.
2. Data awareness
Ensuring awareness across the whole organisation of the key data definitions, widespread awareness of contextual factors, understanding of basic statistical and forecasting principles. This heading could also encompass some elements of the JISC Digital Capability framework, such as maximising organisational knowledge of available technology.
3. Data management
Making sure data definitions are maintained on an ongoing basis, perhaps using a CRUD (Create, Read, Update, Delete) matrix. Also managing links between datasets, holding data owners to account, auditing data quality, considering the data implications of new systems and initiatives, and ensuring all institutional data is available and connectable – not held in silos.
It’s a detailed, unglamorous, analogue set of processes – but essential if you want to unlock digital data capability in your organisation.
AUA Consulting is a consulting practice ‘of the sector for the sector’. AUA Consultants are practitioners in higher education administration and management with a depth of experience and professional expertise. AUA Consulting also offers a team of qualified coaches with expertise in personal, professional and career development.
To find out how AUA Consulting can help you and your team, email consulting@aua.ac.uk. Read more at AUA Consulting.
0 comments on “Unlocking your data capability”